How Child Welfare Workers Reduce Racial Disparities in Algorithmic Decisions
Hao-Fei Cheng
Logan Stapleton
Anna Kawakami
Yanghuidi Cheng
Diana Qing
Zhiwei Steven Wu
Haiyi Zhu
Published at
CHI
| New Orleans, LA
2022
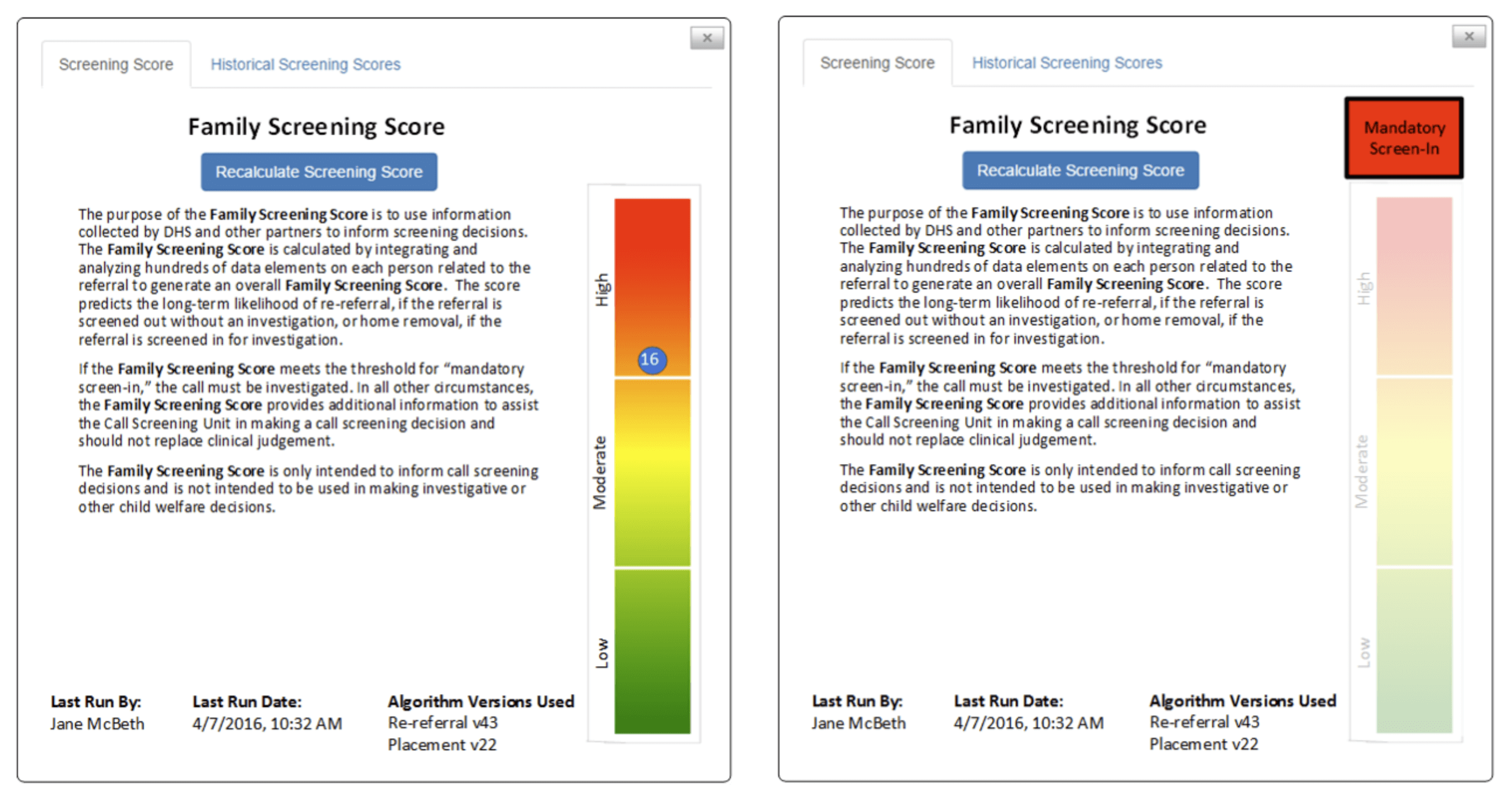
Abstract
Machine learning tools have been deployed in various contexts to support human
decision-making, in the hope that human-algorithm collaboration can improve
decision quality. However, the question of whether such collaborations reduce or
exacerbate biases in decision-making remains underexplored. In this work, we
conducted a mixed-methods study, analyzing child welfare call screen workers'
decision-making over a span of four years, and interviewing them on how they
incorporate algorithmic predictions into their decision-making process. Our data
analysis shows that, compared to the algorithm alone, workers reduced the
disparity in screen-in rate between Black and white children from 20% to 9%. Our
qualitative data show that workers achieved this by making holistic risk
assessments and adjusting for the algorithm's limitations. Our analyses also
show more nuanced results about how human-algorithm collaboration affects
prediction accuracy, and how to measure these effects. These results shed light
on potential mechanisms for improving human-algorithm collaboration in high-risk
decision-making contexts.
Two co-first authors contributed equally to this work.